This first step to AI in Enterprise: Identifying Use Cases, Requirements and ROI
For any enterprise looking to leverage AI, identifying, defining and measuring precisely its use cases, requirements, and potential ROI is a necessary step.
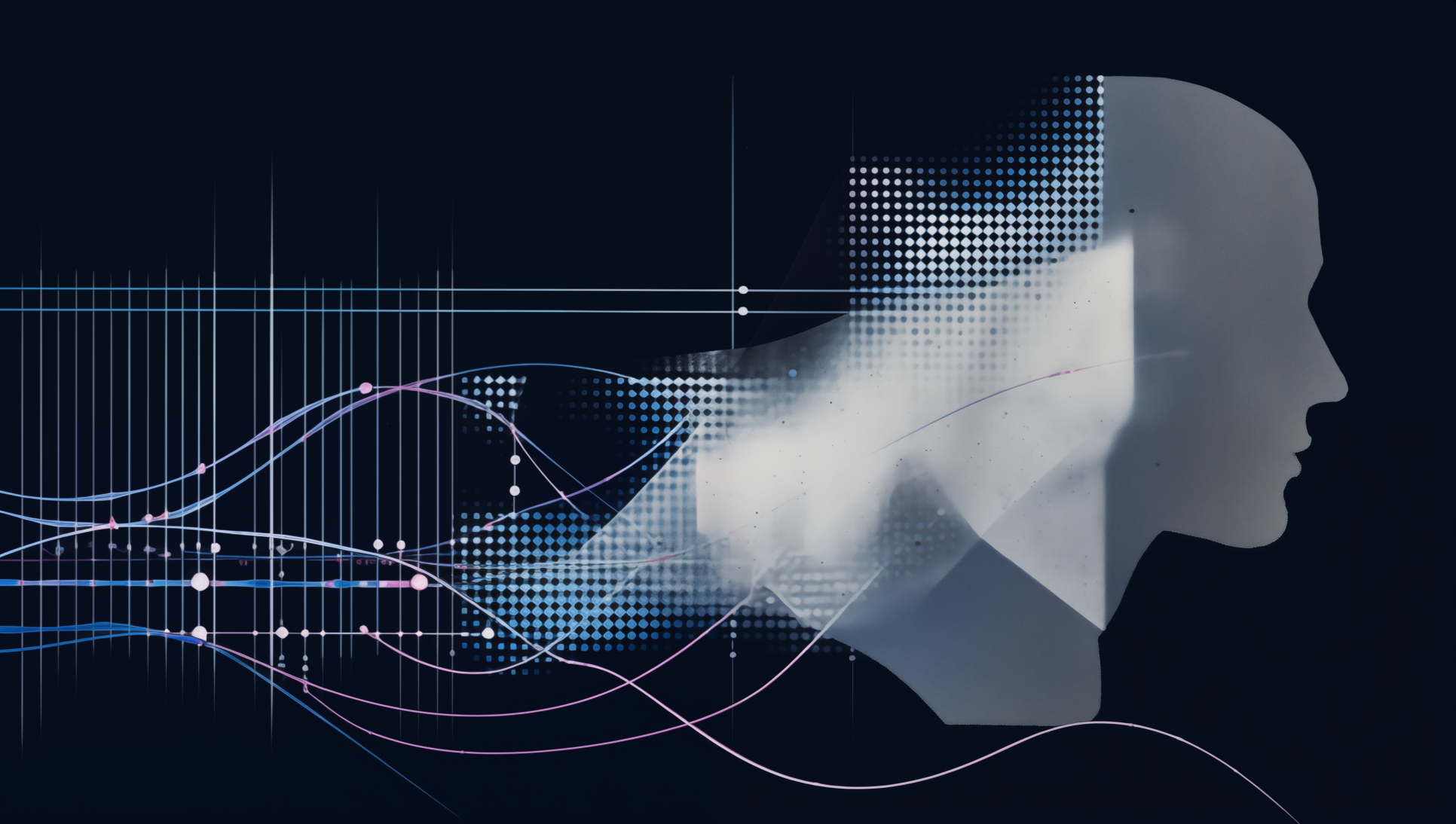
The opportunity presented by artificial intelligence (AI) and generative AI (GenAI) is vast, and the list of potential use cases in production continues to grow as Large Language models (LLMs) continue to improve. New applications for AI are being developed constantly, and unique characteristics in each operating system will generate increasingly bespoke AI solutions and use cases.
With the use of contextual and enterprise-search AI solutions, such as AI conversational chatbots retrieving information, and the rise of autonomous AI Agents, interactions are becoming more sophisticated, and accurate, and GenAI is becoming an invaluable tool to enhance employee's experiences and productivity.
However, for any enterprise looking to leverage AI, identifying, defining and measuring its use cases (i), requirements (ii), and potential ROI (iii) remains challenging.
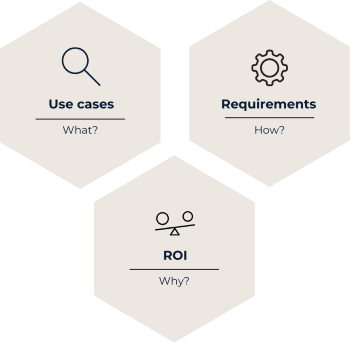
Scoping the use cases
The first step to AI in enterprise is to focus on all the potential use cases, in order to determine where and how AI can infuse efficiency into an organization. Should AI be used to craft fact-based reports? Facilitate enterprise-search? Improve data summarization? Enhance analytics? Or create new business opportunities?
To truly harness AI’s potential use cases, one needs to go beyond the obvious. This involves reimagining the work paradigm potential. A collaborative approach to brainstorming cannot be emphasized enough. Engaging with business leaders, product managers, and AI experts paves the way for a more comprehensive understanding of the potential use cases with impactful results.
The goal at this stage is to understand the potential value creation and efficiency that AI could address into an organization.
Once those use cases are identified, the next step is to identify the use cases in which AI could generate substantial measurable value and contribute to business success relative to the efforts needed to deploy an effective AI solution.
CEOs and executive teams, backed by feedback from employees, need to determine the “golden” use cases — those pivotal areas that promise a competitive edge and internal pain points diminution to free collaborators from painful tasks.
In any case, before you start adopting AI, be sure the use case(s) you have identified might be enhanced by AI, its implications, and technology feasibility. AI will not be magic by itself without a deep commitment, implementation expertise, and acculturation.
The focus should be on selecting the use cases that are high on impact, feasible, have manageable risks and have high ROI. The experience and learnings from these first projects will then guide the further rollout of AI across the organization. You could for example introduce it first for one of your departments, before scaling it to your organization.
Scoping the Requirements
Once the use cases are selected, the next step is to define the AI product's journey from its production to its implementation.
The first question is of course to which extent the AI solution can be developed internally - the traditional question "Build vs Buy". This question simply comes down to "How much can I competitively differentiate if I build versus if I buy?".
Many enterprises are investing in existing tools and platforms, and making these accessible to teams across the enterprise, in order to accelerate the process of moving a use case from ideation to production, and scale the operationalization of AI use cases. This accelerates ideation to production – freeing up more of associates’ time to spend on experimenting and testing, but also helps to democratize AI across the enterprise and building up employees' skill sets.
However, if you can of course use proprietary models and API, such as ChatGPT, it poses important privacy, compliance and security concerns, its usage might not fit your specific use cases, and it might be challenging to scale considering it's not malleable, nor cost-predictive. This is why it should be limited to quick POC test.
In any case, any enterprise dealing with sensitive information should caution employees against using public chatbots, and consider using commercially available LLMs and hosting them (either on a dedicated private cloud or through on-premise deployments). If such a deployment might be more expensive (which is not always the case), it brings a new level of security, confidentiality, but also personalization, enabling you to match your development with your use cases (in particular over-time).
Creating its own roadmap calls for another round of collaboration. Technical and business leaders, supplemented by AI experts, converge to assess critical factors. Depending on the use cases, some important topics should be discussed, notably about:
- the hosting of the solution,
- the choice of the large language model (LLM),
- the extent of model customization,
- the evolutivness of the AI solution (regarding the latest LLMs),
- data utilization, storage, and control,
- employees access and training (how do I coach my employees to ask the right questions to get the best output?),
- compliance measures,
- an evaluation of the extended costs, notably for maintaining AI projects over the long-term (which implies a predictive pricing model matching a scalable deployment),
- etc.
This phase often highlights the need for external expertise. Investing in external experts, especially in niche areas like model fine-tuning, can be the difference between a successful and a mediocre off the shelf AI implementation. In order to get good outputs, you need to create a data environment, involving data engineering skills and machine learning capabilities.
Many organizations find that they don't have ready-to-use data (unstructured data is very common), making external assistance, both in terms of manpower and tools, invaluable for tasks like data curation – the process of organizing, cleaning, annotating, and enhancing raw data, to enhance AI outputs.
Measuring the Return on Investment (ROI)
It’s important that you can measure and prove the success of your AI project, so decide early on the key performance indicators, and how to assess the tangible impact of AI on the organization. Every use case should produce measurable outcomes (income uplift, cost reduction, task execution time, customer satisfaction, reallocation of resources, creation of new opportunity, employee or customer satisfaction, or even some indicators related to the happiness at work).
However, it is worth noting that determining ROI for AI and GenAI solutions proves to be more intricate than with traditional tech investments. Assessing the specific contribution of AI investment — like profit boosts or cost reductions — is often challenging. Decision-makers may need to rely on estimates rather than concrete values. For many use cases, there may not be adequate available information, and a hypothesized approach may be required.
Additionally, the derived value from AI may not consolidate into a singular figure, but may be distributed across various departments and teams. AI use cases can have multiple impacts outside the primary purpose. For example, an AI tool that retrieves knowledge for employees may drive value in terms of talent retention and training.
ROI for AI typically extends to the long haul, often spanning more than a few months. Unforeseen obstacles — ranging from data access issues to challenges in model training — can prolong project timelines. AI projects may occasionally face setbacks, pushing teams to reboot their efforts.
At Lampi, we securely provide an AI-powered platform with the best and latest LLMs to perform entire workflows thanks to predictable and fine-tuned AI agents that are trained to perform identified key use cases with high added value and representing repetitive tasks specific to several industries.
Ready to transform how you work with AI? Contact us to request a demo.
Don't forget to follow us on LinkedIn, Twitter, and Instagram!