The Limits of Using Large Language Models (LLMs) in your Enterprise
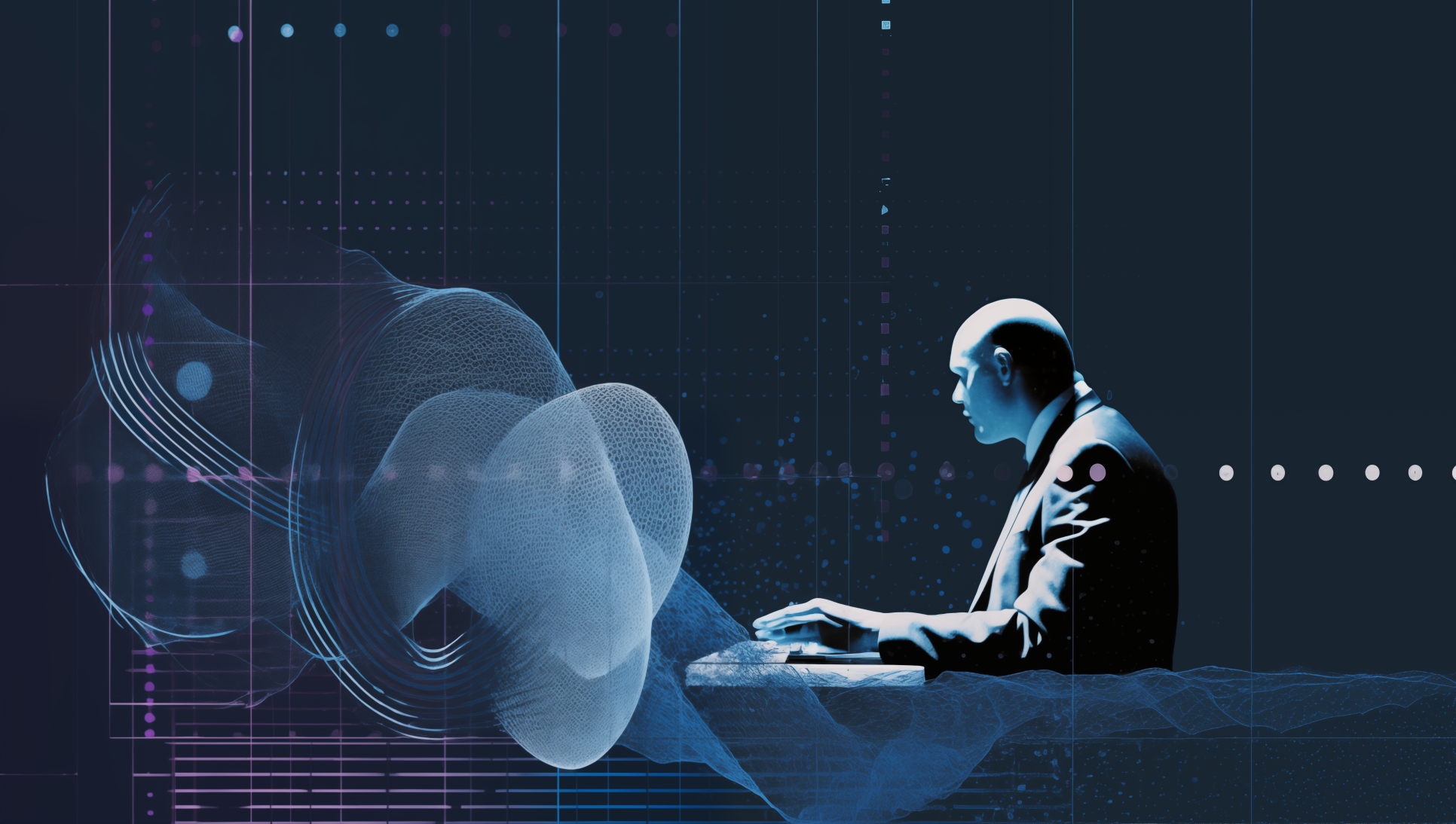
In a business context, Large Language Models (LLMs) – used as a copilot for example – have the advantage of understanding any query and providing natural language relevant and helpful answers – just like any other colleague.
Just think about ChatGPT. It is more likely that most of your employees are already using Generative Artificial Intelligence (GenAI) in their daily business tasks thanks to the natural language processing capabilities of LLMs that offer unprecedented speed, and efficiency, mimicking a human-like colleague that can assist in a wide range of tasks.
While these models bring forth an exciting realm of possibilities—streamlining operations, enhancing customer service, and driving innovation—they are far from being a silver bullet. In fact, their integration into a business ecosystem is not without hurdles and limitations.
More precisely, simply applying these models to your business might involve:
- An important lack of security, privacy, and confidentiality with no access controls: your raw data or data flows might be used for data training, read, or shared with third parties. As such, many companies have banned chatbots inside their businesses because of the risk that sensitive data or customer data will be leaked outside the company. For illustration, Amazon warns employees not to share confidential information with ChatGPT after seeing cases where its answer “closely matches” existing material from inside the company. Samsung Electronics Co also banned employee use of popular GenAI tools like ChatGPT after discovering staff uploaded sensitive code to the platform, dealing a setback to the spread of such technology in the workplace. Apple, and JPMorgan also ban the use of public Generative Tools including LLMs like ChatGPT, Bard, etc.
- An absence of advanced domain-specific knowledge and context – If you ask a generic LLM a question about the last trend or your last deal, it will likely tell you that it doesn’t have enough context to answer. Worse yet, it might hallucinate and fabricate an incorrect reply, resulting in the spread of misinformation and potentially serious workflow consequences. If “prompt engineering” can help to get better output text by providing additional context to the model (such as by giving a few high-quality examples or some information), this solution has its limitations in terms of efficiency and confidentiality.
- A risk of nonrelevant outputs with an increased risk of misuse and biases due to missing explanations and transparency, or hallucinations from combining unrelated sources to create new “facts.”
For enterprises to reap the benefits of LLMs and integrate GenAI as part of their strategy, they must overcome these challenges, which raise important questions about the readiness and appropriateness of LLMs for specific business applications. How secure are they? How accurate and context-aware can they be?
Regarding the latest point, to improve the contextual accuracy of outcomes, it’s important to be able to connect models with your data. While using Application Programming Interfaces (APIs) to connect these models is a quick solution for some enterprises, it comes with cons and challenges, particularly in terms of security, data noise, data quality, prompt efficiency, output quality, and long-term effectiveness.
Fine-tuning a large language model, which refers to the process of adjusting and adapting a previously trained model to better handle certain tasks or to be specialized in a particular domain, is generally presented as a solution to integrate data with a model. However, and regardless of the costs it involves, such a solution has its own limits:
- Permissions: in any organization, it's vital to ensure controlled access to company data. This control is merely impossible when a LLM is at the disposal of all your employees, and you wouldn't want employees to retrieve sensitive data by merely posing questions to the model;
- Explainability: when using GenAI on data, enterprises expect of course to get accurate answers, but it’s also important that the information provided by the model is verifiable. For example, if a financial analyst receives investment advice from an AI copilot, it is always better to be able to check the reference of the original report or study backing that recommendation. Blindly relying on LLM outputs doesn't allow for such validations.
- Up-to-date data: it is crucial that the LLM can access continuous data to retrieve all the necessary information and context (which would require retraining the model regularly). Indeed, when you think about an AI copilot for your enterprise, you expect to engage with your AI copilot (chatbot) to receive swift and up-to-date answers to your inquiries, sidestepping the process of combing through extensive search result pages.
Those are just some limits that highlight the limits of fine-tuning with enterprise's data and the need to implement a search system that feeds LLMs with verifiable data to produce content (i.e., Retrieval Augmented Generation or RAG).
In our coming publications, we will present in detail the implications of fine-tuning and how to implement such search solutions.
In any case, the incorporation of LLM into a business requires a careful evaluation of both the extraordinary opportunities and the inherent challenges that these models bring.
At Lampi, we're passionate about enabling businesses to fully leverage the potential of AI.
Lampi is a confidential and collaborative AI copilot that empowers your workforce in their business tasks.
Lampi safely brings an AI-powered platform to conduct extensive searches across your entire knowledge and helps you with quality content generation, brainstorming, and much more based on your knowledge.
Our experts are always ready to guide you on your AI journey, helping you understand and navigate the complex world of AI.
So, why wait? Step into the future with Lampi and embark on your AI journey today!
Don't forget to follow us on Twitter, Instagram, and LinkedIn!