Choosing the Right AI Solution for Your Business: A Practical Overview
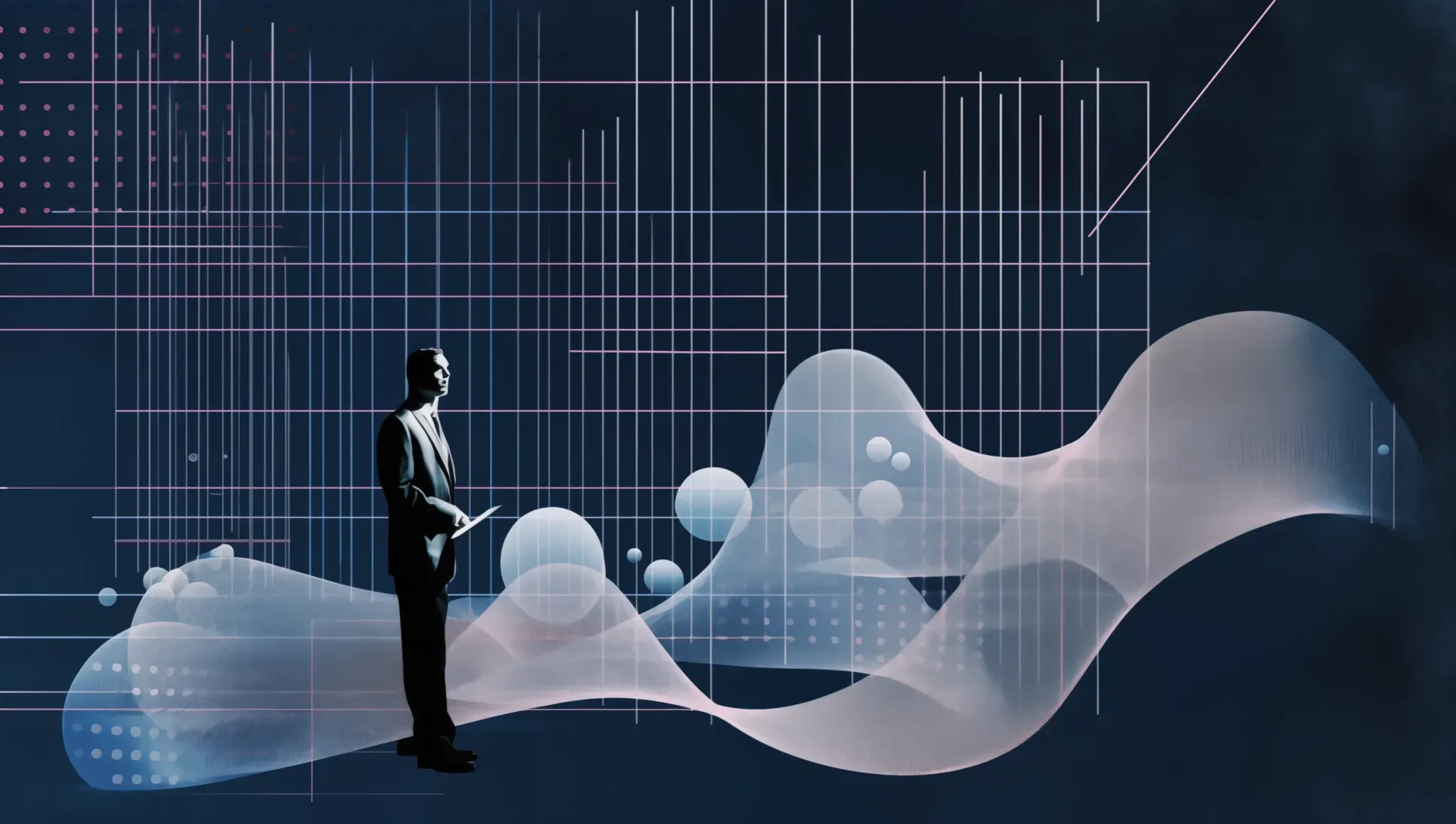
The time when AI-powered solutions were reserved for a small part of organizations with important resources and highly skilled team is over. Today, almost any organization can decide to apply Artificial Intelligence (AI) or Generative Artificial Intelligence (GenAI) to their business to unlock unprecedented efficiencies and innovations.
If the hype is real, as almost every CEO has begun adding AI to their business strategy, there are a lot of considerations to think about when implementing an AI solution in your organization, in particular privacy and security concerns, and the journey to effective incorporation is not always straightforward. While there's no single correct approach to integrating AI, the choice of how to implement AI within your business often hinges on various factors such as budget constraints, the expertise of your team, specific use cases, and many other considerations.
Although there's no one-size-fits-all strategy, the purpose of this article is to provide an overview of existing pathways to implement an AI solution. Our goal is to demystify the intricate maze of these decisions and to offer insights into the different options for businesses embarking on their AI journey to choose the right one.
Building its own Model
Opting to build your AI model can promise world-class performance and provides greater control, but this endeavor comes at a significant cost (millions of dollars).
Beyond monetary costs, businesses need to factor in the requirements for data centers, advanced computing infrastructure, and skilled talent to train and deploy a model. The journey to establishing an enterprise-grade model isn't swift.
Several sequential steps need unfolding, and while some processes can run concurrently, they can't be entirely parallelized:
- Data Collection: Accumulating the right set and volume of data is a lengthy process, ranging from weeks to several months.
- Talent Acquisition: Hiring the right data scientists skilled in LLM, or even onboarding consultants with the necessary expertise, is a process that could span weeks to months.
- Training & Deployment: Once you have the data and talent in place, the model has to be trained and subsequently deployed, which requires an experienced skilled team and an important infrastructure to handle it.
- Integration: The model needs seamless integration into existing business systems for practical utility, which also has its challenges.
As such, creating a foundational model from scratch is generally considered as beyond the means of many organizations. Most companies will likely rely on third parties, either by leveraging open-source models or through API models (e.g., Anthropic’s Claude or OpenAI’s GPT-4), which can allow seamless and more cost-effective integration.
Leveraging pre-trained and public models
The easiest and fastest method to implement AI is of course to use an existing foundational model – like OpenAI's GPT-4 and Anthropic's Claude, which are incredible at generating coherent and contextually relevant text based on given prompts and can assist in a wide range of tasks, such as writing, translation, and even conversation.
You can use APIs from companies like OpenAI or Anthropic where you submit your prompt, get a response, and pay usage based fees.
How we ask for something has a major effect on the quality of the output that we get. You need to be precise, and complete and give examples of the output you are interested in. This prompt method is called “prompt engineering”, the technique of getting a model to perform tasks directly by inputting prompts without training it on particular data and/or expected outputs. Prompt engineering includes different techniques such as zero-shot prompting, single-shot prompting, or few-shot prompting (with examples).
This pathway comes with higher security risk, lower quality performance at domain-specific tasks, and less control over the underlying models. Using API means you are a taker of whatever third parties are offering. Model features, customizations, and values are all dictated by those companies. You can only build a front-end.
Leveraging open-source models
Another way to implement GenAI is to use and leverage open-source Large Language Models (pre-trained models available to the public). Open-source LLM is a perfect solution to leverage LLMs without disclosing data by storing open-source LLMs on-premises or on a dedicated server.
However, while LLMs are indeed powerful and capable of remarkable feats, they often require a process known as 'fine-tuning' to achieve their maximum efficacy. Indeed, models are great generalists, but for enterprise use cases, they often fall short as specialists, particularly when there's a need for domain-specific or company-specific information.
Fine-tuning a large language model refers to the process of adjusting and adapting a previously trained model to better handle certain tasks or to be specialized in a particular domain. It generally helps with domain-specific knowledge, contextual understanding, and bias reduction.
The biggest challenges for fine-tuning foundation models are acquiring training data and the necessary infrastructure.
In any case, both of these pathways have specific limitations, for example:
- The amount of data they have access to: if you want to add up-to-date data, you need to retrain the model. This limitation affects the model's ability to generate up-to-date and accurate responses.
- The potential lack of expertise (except in the case of fine-tuning or with specific models): LLMs are trained on a large dataset that covers a wide range of topics, but they are not specialized in a specific domain knowledge, which can increase hallucinations or provide inaccurate information.
- They lack of explainability. LLMs don't have a reliable way of returning the exact source of their answers, which makes it impossible to verify. This exacerbates the issue of hallucination, as they may not be able to provide proper attribution or verify the accuracy of their responses.
This is why, a new solution emerged, as a pipeline between LLMs and your data.
Retrieval Augmented Generation (RAG)
When using GenAI with your data, it is crucial that the LLM can access continuous data to retrieve all the necessary information and context. Indeed, when you think about an AI copilot for your enterprise, you expect to engage with your AI copilot (chatbot) to receive swift and up-to-date answers to your inquiries, sidestepping the process of combing through extensive search result pages. Additionally, it is always better to get citations for users to fact-check or delve deeper into the information provided by the model.
A viable remedy for this concern is the incorporation of a search system that feeds LLMs with verifiable data to produce content. Retrieval Augmented Generation (RAG) is a data augmentation technique using semantic embeddings, that retrieves data from outside a foundation model and augments your prompts by adding the relevant retrieved data in context.
It works by connecting to a vector database and fetching only the information that is most relevant to the user’s query. Using this technique, the LLM is provided with enough background knowledge to adequately answer the user’s question without hallucinating. RAG is not a part of fine-tuning, because it uses a pre-trained LLM and does not modify it in any way.
It can present the following advantages:
- Updated data: Knowledge remains constantly updated and pertinent, given that the LLM is consistently fed with a current search index during each query. Users have always access to the latest data (connected), which cannot be forgotten among the vast amount of parameters of LLMs,
- Verification: For verification purposes, users can review the specific documents provided to the LLM, ensuring that its responses are based on factual information. The model sidesteps the issue of catastrophic forgetting, as it pulls the necessary knowledge in real time instead of attempting to internalize all information,
- Permissions: RAG can be designed, so the LLM refrains from accessing any data outside a user's permission.
Preparing for the Journey Ahead
Each of these approaches has its own set of advantages and disadvantages, and it's imperative to weigh them carefully. Security, costs, complexity, and the scale of deployment are among the many factors that will influence your choice.
Embarking on the GenAI journey could be one of the most transformative decisions for your business. However, success necessitates a well-thought-out strategy that considers all variables, from costs and technical requirements to human capital and long-term sustainability.
At Lampi, our mission is to accelerate the development of AI applications for enterprises. That’s why we are excited to share our knowledge and best practices for organizations to deploy AI for real business impact.
Discover more about our AI solutions on our website
Don't forget to follow us on LinkedIn, Twitter, and Instagram!