AI for Business: the importance of curating High-Quality Data
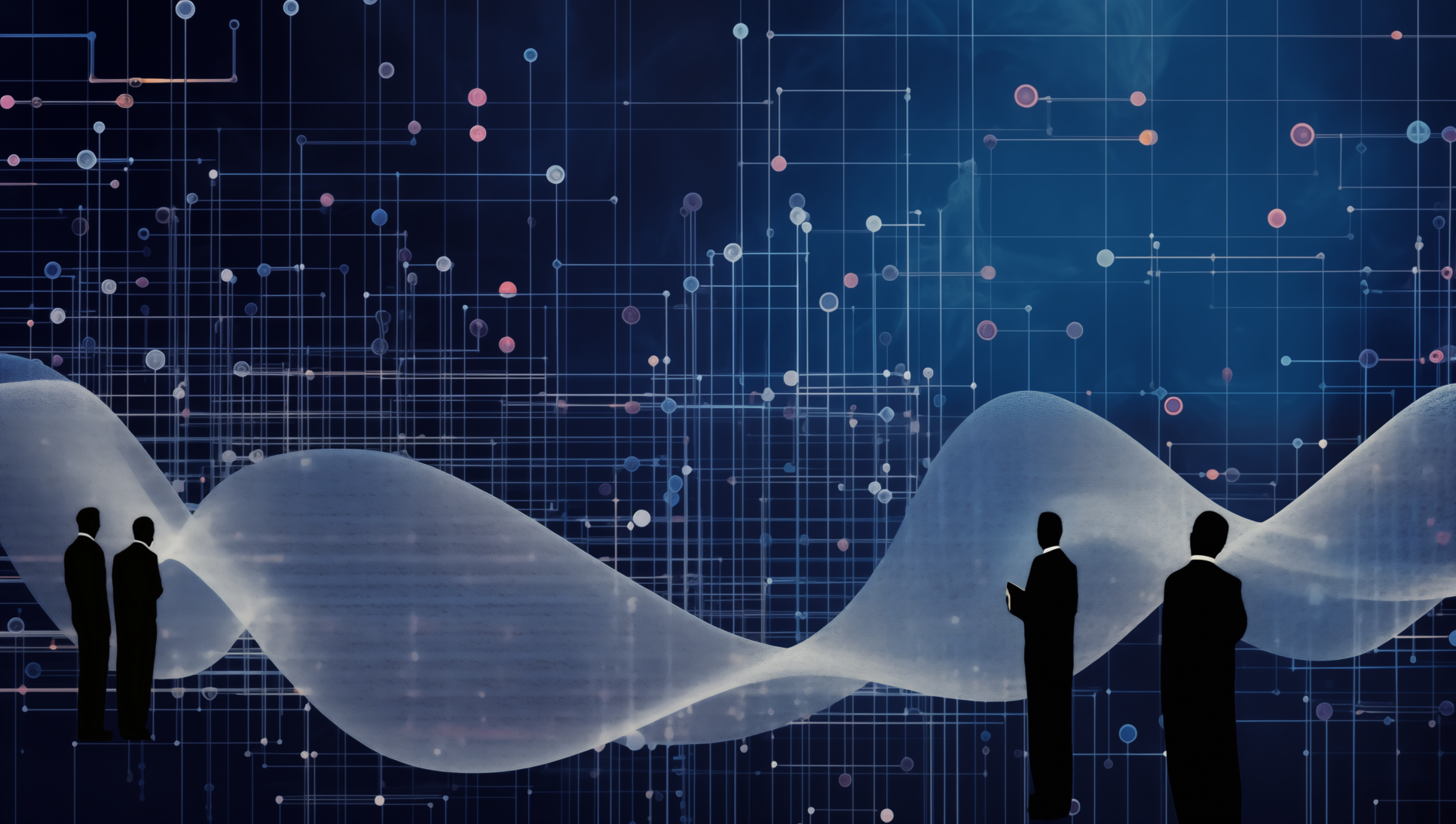
Artificial Intelligence (AI) and Generative AI have recently emerged as a game-changer for business, taking innovation, efficiency, and productivity to a new level.
Using AI has become accessible to everyone, and large language models (LLM) have been opened to anyone willing to use it. However, if you want to leverage AI efficiently in your workflow, it is necessary to use AI with your unique data.
New tools have emerged and are offering users the ability to ask questions, receive contextually relevant answers, and generate insights directly from the knowledge they provide themselves.
This is what is proposed by Lampi, a confidential AI copilot designed for business, which allows you to seamlessly integrate your knowledge (from documents and applications) and fully unlock AI's capabilities.
How does it work?
Without going into too much detail (an article will be published on this specific topic), using AI on your data is permitted through a specific process
First, text extraction (e.g., from documents or websites), where all textual content from a user-provided document is extracted for further steps.
This information is subsequently partitioned into smaller segments, called 'chunks', with a predetermined character count, ensuring the text is primed for deeper analysis.
The process known as 'embedding' follows, generating multiple numerical representations or 'embeddings' for each text chunk. Embeddings are one of the most versatile techniques in machine learning. Embeddings are a way of representing data–almost any kind of data, like text, images, videos, users, music, whatever – as points in space where the locations of those points in space are semantically meaningful.
Every individual text chunk undergoes a thorough analysis with identical descriptors, culminating in the formation of a 'vector.' This vector, a comprehensive array of numbers, serves as a distinctive identifier, much like a unique fingerprint for each text chunk. All embeddings are then meticulously cataloged in a 'vector database' ready for future search queries. When a user asks a question, it's also converted into a vector.
The transformation allows the AI system to perform an accurate 'semantic search' by comparing the vector of the asked question to the vectors of pre-existing text chunks. The system (when it is well designed) identifies and retrieves text chunks that are most closely related to the asked question. These related chunks are then employed to generate a 'prompt,' which guides the AI tool in crafting a precise response, thoroughly contextualized to the user's query.
This technical process is however useless without one important step: the curation of the data you upload.
The Primacy of Quality Data
To truly benefit from AI, the quality of the input data is paramount. AI models are only as good as the data they're trained on. A tool might boast advanced algorithms and innovative features, but without well-curated and contextually accurate data, these advantages amount to little. It’s similar to a chef who needs quality ingredients to cook a sumptuous meal. Even the most skilled chef can't compensate for subpar ingredients. Likewise, even the most advanced AI models can't make up for poor-quality data.
So, in the quest for AI-enabled success, remember the vital role of quality data and choose tools that truly put your data's potential into action.
The performance, accuracy, and effectiveness of an AI system are largely dependent on the quality and relevance of the data it is trained on. Therefore, data curation — the process of organizing, cleaning, and enhancing raw data — becomes an indispensable step.
High-quality data leads to precise outputs, enabling better decision-making and forecasting.
As such, when integrating knowledge, data from different documents or applications must be gathered, cleansed, and carefully selected to draw out valuable insights. Irrelevant or redundant data can cloud the AI model's decision-making process and affect its ability to perform tasks or make accurate predictions.
After data curation comes the vital step of data integration. It involves combining data from disparate sources into a unified, coherent view. Integrating your data allows for a comprehensive understanding of your business operations, as it breaks down data silos and provides a complete picture.
Key steps to curate high-quality data involve notably:
- determining what quality data means in the context of your specific business requirements. This definition will differ across sectors and individual businesses;
- identifying relevant data sources: seek out sources that can provide the quality of data you require. Ensure these sources are reliable and consistent.
Conclusion
Implementing AI in business operations is no longer a futuristic concept but a present-day reality that, when executed correctly, can yield considerable benefits. However, this journey demands a meticulous, step-by-step approach.
With Lampi, you can not only apply AI to your data, but you also gain a partner in data curation and selection, essential steps towards obtaining accurate AI outcomes.
Lampi’s no-code AI solution makes implementing AI in your business easy and quick. Lampi can help you develop flows that deliver accurate results exactly where you need them, whatever your activity or expertise.
Don't forget to follow us on Twitter, Instagram, and Linkedin!